
Invited Speakers & Panelists
Title: Applications of deep learning to critical obstacles understanding and treating neurodegenerative disease
Start Time: 8:45 AM
Speaker: Dr. Steve Finkbeiner, Taube/Koret Center for Neurodegenerative Disease Research at Gladstone, Roddenberry Stem Cell Center, University of California, San Francisco
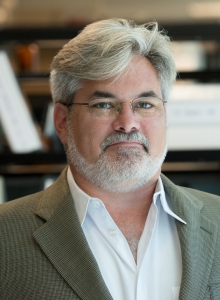
Abstract: How is it possible that we still have nothing to offer patients with devastating incurable diseases like ALS, autism, Alzheimer’s disease, schizophrenia and Parkinson’s disease even though we have known about these diseases for over a century in some cases? Do the advances in computer vision have anything to offer to help?
In this talk, we will introduce the challenges and opportunities in the area of neurodegenerative diseases, emphasizing some of the obstacles where computer vision can help. To address some of the challenges, we built automated microscopes and developed patient-derived stem cell models of human neurodegenerative diseases. In addition, we developed an array of several hundred biosensors to expand the feature set from images. These methods generate large highly multidimensional imaging datasets amenable to analysis with supervised and unsupervised machine learning, which we are using to better understand the causes of disease, to find targets that can be pursued therapeutically, and to investigate the structure and nature of patient populations, which could lead to stratifications that could help make clinical trials more successful.
Bio: Dr. Finkbeiner is best known for his pioneering work on brain disorders. He invented robotic microscopy, a new form of imaging that has helped unravel cause-and-effect relationships in amyotrophic lateral sclerosis (ALS, or Lou Gehrig’s disease), Huntington’s, Alzheimer’s, Parkinson’s, autism and schizophrenia. Dr. Finkbeiner used this technology to resolve a long-standing puzzle related to one disease, and a study based with this approach became the most-cited paper in the field of neuroscience of the last decade. The scope and scale of data produced with this approach has enabled the application of deep learning to address some of the critical obstacles to understanding and treating these brain diseases, including disease modeling, early diagnosis, patient stratification, and therapeutic discovery.
Title: Mapping the brain at cellular resolution: academic and pharmaceutical industry applications
Start Time: 9:45 AM
Speaker: Dr. Pavel Osten, Cold Spring Harbor Laboratory and Certerra
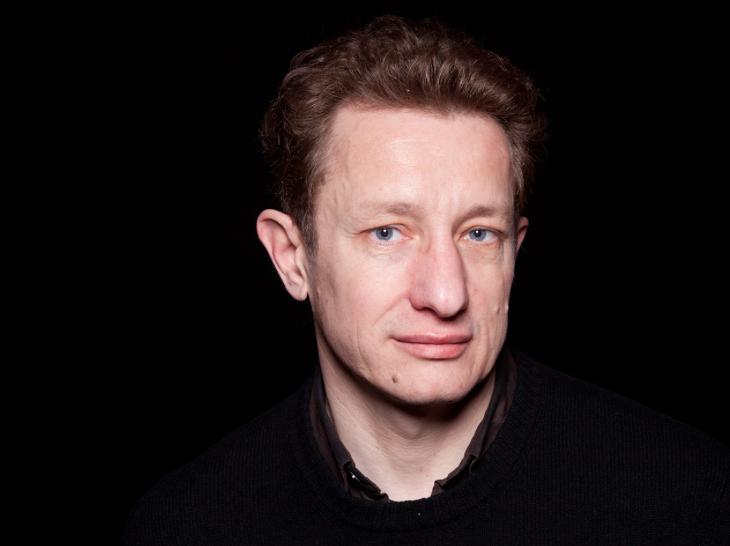
Abstract: The beginning of the 21st century has seen a great renaissance in light microscopy in neuroscience, with new instruments and brain clearing and staining methods capable of generating complete datasets for the mouse brain at up to a submicron brainwide resolution. Here I will review the main pros and cons of the different types of the microscopy instrumentation and methods, and I will also describe our computational pipeline and toolchain software called Headlight for reconstruction, anatomical registration and computational analyses of the whole brain datasets. Finally, I will give examples of scientific applications from my lab at CSHL in systematic atlasing of cell-type distribution and morphology as well as from a biotech start-up Certerra focused on commercializing these methods for CNS drug screening.
Bio: Dr. Pavel Osten received his MD degree from the Medical School of Charles University, Prague, Czech Republic, and his Ph.D. from the State University of New York, HSCB. He is currently Associate Professor at the Cold Spring Harbor Laboratory and founder of Certerra, a company that is working at the interface of systems neuroscience and automated microscopy, and knowledge. His research interests include the development of viral vector-based methods for gene therapy applications, with a particular focus on targeting of cell type-specific neurons and identified neuronal networks, and the study of gene and cellular functions underlying the contributions of individual neurons and defined networks to cognitive performance. In 2005, he was the recipient of a Wellcome Trust Senior Fellowship.
Title: Industry/Government Panel on Research Priorities and Funding Opportunities
Start Time: 11:30 AM
Panelists: Dr. Matthew Andrew(ZEISS), Dr.Peter Bajcsy(NIST), Dr.Lawrence W.C. Chan(PolyU), Dr.Michelle Freund(NIH), Dr.Daniel Hoeppner(Astellas), Dr.Jie Yang(NSF)
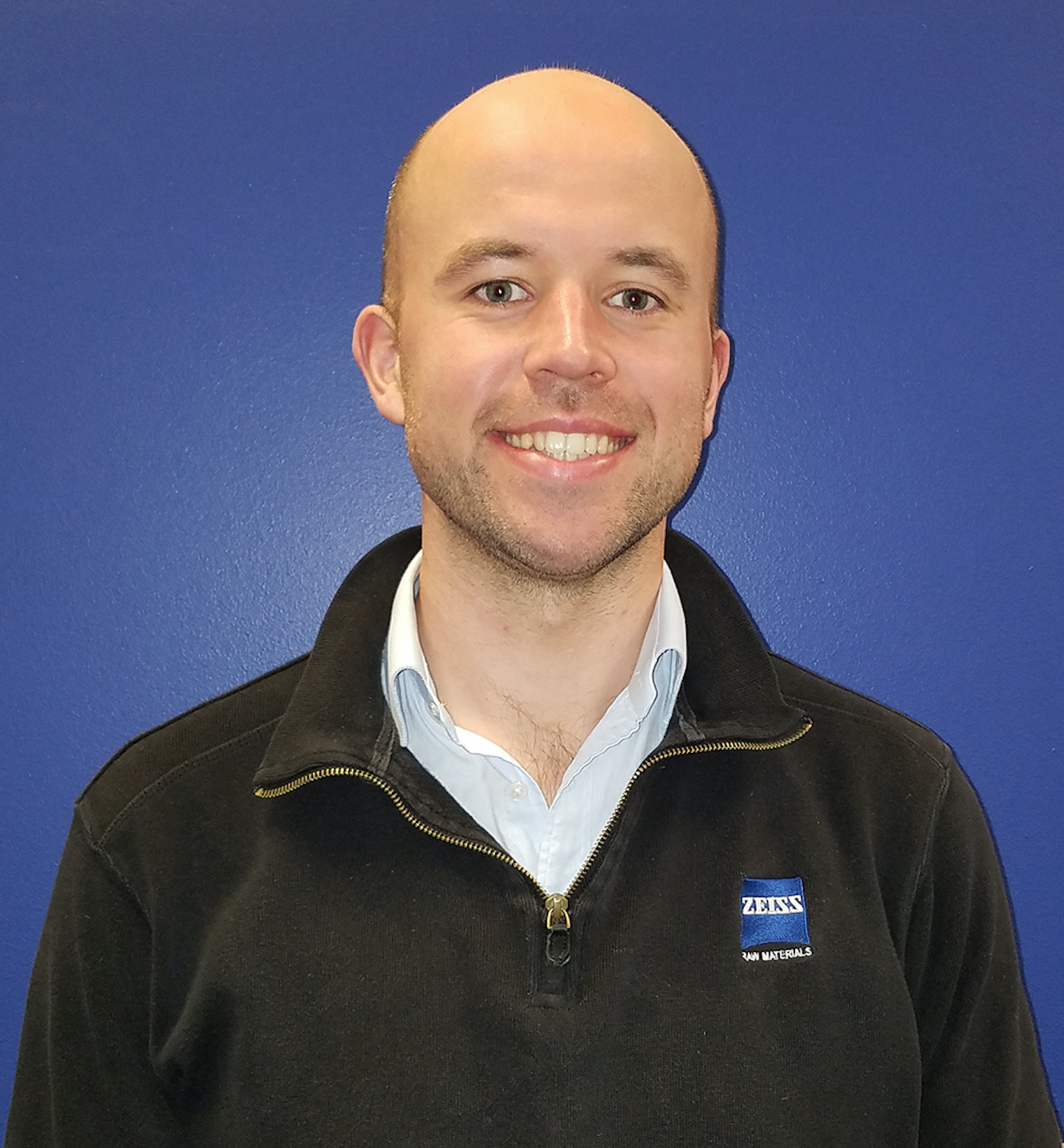
Dr. Matthew Andrew currently directs development of high resolution imaging technologies for the Oil and Gas Industry and the Geosciences within ZEISS microscopy. He completed his PhD at Imperial College London, where he developed the first pore scale core analysis system capable of imaging multiple fluid phases at reservoir conditions, which he used to examine a range of petrophysical properties including wettability, capillary pressure, flow dynamics and trapping behavior. His current research interests include the use of multiscale experimental methods for the investigation of multiphase flow, the integration of multimodal high resolution analysis with modern data science tools, the application of automated high resolution imaging technology to petroleum operations problems and the integration of data science techniques with petroleum production.
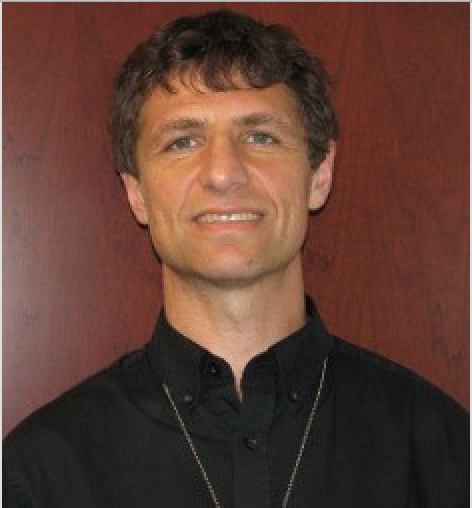
Dr. Peter Bajcsy received his Ph.D. in Electrical and Computer Engineering in 1997 from the University of Illinois at Urbana-Champaign (UIUC) and a M.S. in Electrical and Computer Engineering in 1994 from the University of Pennsylvania (UPENN). He worked for machine vision, government contracting, and research and educational institutions before joining the National Institute of Standards and Technology (NIST) in 2011. At NIST, he has been leading a project focusing on the application of computational science in biological metrology, and specifically stem cell characterization at very large scales. Peter’s area of research is large-scale image-based analyses and syntheses using mathematical, statistical and computational models while leveraging computer science foundations for image processing, machine learning, computer vision, and pattern recognition. He has co-authored more than more than 39 journal papers and 11 books or book chapters, and close to 100 conference papers.
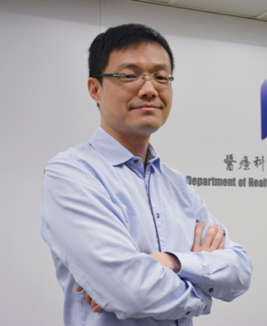
Dr. Lawrence W.C. Chan received his PhD in Artificial Intelligence in 2001 from the University of Hong Kong. Dr. Chan is currently Associate Professor in the Department of Health Technology and Informatics, the Hong Kong Polytechnic University. He has been appointed as Editorial Board Member, Engineering Applications of Artificial Intelligence (EAAI); Associate Editor, Frontiers in Non-Coding RNA; and Affiliate Member, Hong Kong Society of Medical Informatics (HKSMI). Dr. Chan’s research focuses mainly on artificial intelligence, biomedical big data, medical imaging, and genomics. His recent research findings have been published in international journals, such as Scientific Reports, Oncotarget, Biochimica et Biophysica Acta, and resulted in 2 patents. Because of his outstanding academic performance, Dr. Chan received the Silver Award in “the 53rd World Exhibition of Innovation, Research and New Technology” and the Certificate of Merit, “Radiological Society of Northern America”. Dr. Chan, as the project leader, completed a series of research projects supported by competitive research grants, including General Research Fund (GRF: PolyU 5118/11E) for his project about EHR Clinical Decision Support System, and Health and Medical Research Fund (HMRF 02131026) for his project about targeted therapy resistance in lung cancer (two projects: HK$1.78 million in total). Dr. Chan has established the Biomedical Big Data Laboratory in Hong Kong Polytechnic University supported by the sponsorship of Huawei Hong Kong to jointly develop scientific research projects covering AI pathology.
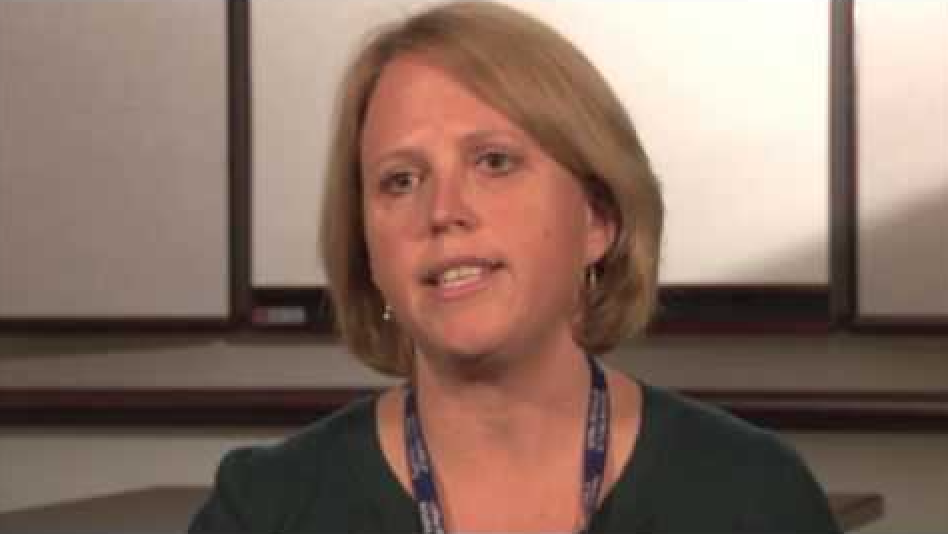
Dr. Michelle Freund is Scientific Program Coordinator in the Office of Technology Development and Coordination at the National Institute of Mental Health, NIH, Bethesda, MD. As a program officer, she manages a research portfolio of grants that are focused on the development of novel tools and technologies important for the advancement of basic and translational neuroscience. Michelle serves as the Director for the NIH NeuroBioBank, a network of six brain and tissue repositories that provide post-mortem human brain samples for research. She is an active member of several trans-NIH interdisciplinary teams such as the NIH BRAIN Initiative and the Blueprint for Neuroscience. As co-lead on a BRAIN Initiative team, she provides guidance and oversight for the Cells and Circuits focus area outlined in the BRAIN 2025 report. Michelle received a B.A. from the University of California, San Diego in mammalian physiology and a Ph.D. in Neuroscience from Hahnemann University in Philadelphia. Before joining NIH in 2007, she studied the role of monoamine neurotransmitters in the actions of antidepressant drugs and the interactions of stress and drug addiction.
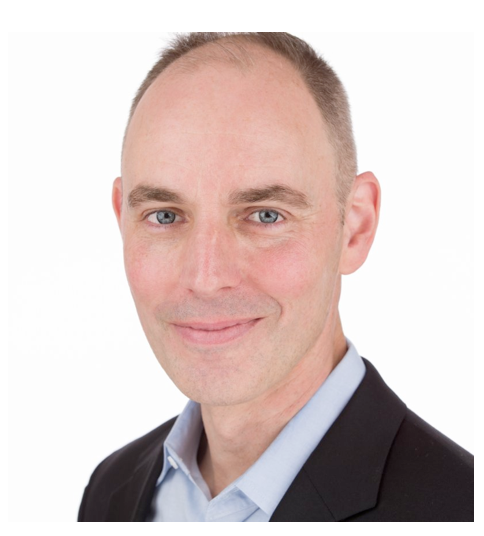
Dr. Daniel Hoeppner is an Associate Director of Neuroscience at Astellas Research Institute of America (ARIA), in San Diego CA. He manages a diverse team of scientists focused on identifying new therapeutic interventions for patients suffering with psychiatric illness. His team applies the tools of human genetics, next-generation sequencing, human iPSC (stem cell) technology, mouse models, and automated microscopy to identify and validate novel targets for intervention. Prior to Astellas, Dr. Hoeppner was an investigator at the Lieber Institute for Brain Development (Johns Hopkins, Baltimore MD), a Staff Scientist at the NIH-NINDS (Bethesda MD), and a graduate student of genetics at Cold Spring Harbor Laboratory (Long Island, NY).
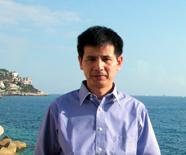
Dr. Jie Yang is a Program Director in the Division of Information and Intelligent Systems (IIS) at the National Science Foundation (NSF). He is the leader of the Robust Intelligence Cluster and oversees computer vision research. He has also been involved in many other crosscutting programs. Before joining NSF, he was a faculty member in the School of Computer Science at Carnegie Mellon University, where he worked on multimodal/multimedia, computer vision, pattern recognition, robotics, and automatic control. He was an Advisory Board member of the ACM International Conference on Multimodal Interfaces (2003-2015). He also served as Program Co-chair (2002) and General Co-chair (2006 and 2010) for ACM International Conference on Multimodal Interfaces, as well as a Program Co-chair (2010) for ACM Multimedia. He served as a General Co-chair (2014) for the IEEE International Conference on Multimedia & Expo. He served as an Associate Editor for the IEEE Transaction on Multimedia (2004-2008). He is an Associate Editor for the Machine Vision Applications journal. He also served as Area Chair and Program Committee member for multimodal/multimedia, computer vision, and pattern recognition conferences. He is a fellow of IEEE.
Title: Assessment of intra-tumor heterogeneity and evolutionary trajectories in cancer
Start Time: 13:30 PM
Speaker: Dr. Subhajyoti De, Rutgers, the State University of New Jersey and Rutgers Cancer Institute
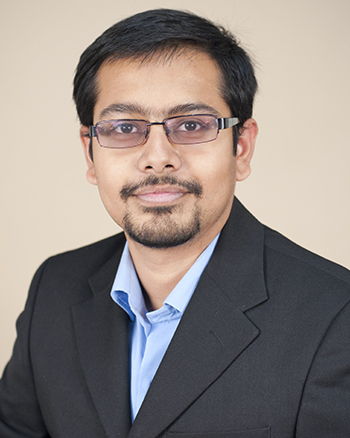
Abstract: Nuclei segmentation plays an important role in digital pathology image analysis as the accurate separation of nuclei is crucial for cancer diagnosis and other clinical analysis. Current learning-based methods in nuclei segmentation have certain limitations in lowering localization accuracy. We develop FullNet, a full resolution convolutional neural network that achieves good performance in nuclei segmentation. Using annotated histopathological images, we show the effectiveness of this approach in nuclei segmentation and compare it to other state-of-the-art methods. We then describe our initiatives to integrate histopathological imaging and genomic data to inform about intra-tumor heterogeneity and evolutionary trajectories in cancer. Analyzing histopathological images for 55 BRCA1-associated breast tumors, we assess the status of BRCA1, PTEN, and p53 mutations at the single cell level. We then use computational methods to predict the relative temporal order of somatic events, on the basis of the frequency of cells with single or combined alterations. Although there is no obligatory order of events, we find that loss of PTEN is the most common first event and is associated with basal-like subtype, whereas in the majority of luminal tumors, mutation of TP53 occurs first and mutant PIK3CA is rarely detected. We also observe intratumor heterogeneity for the loss of wild-type BRCA1 and increased cell proliferation and centrosome amplification in the normal breast epithelium of BRCA1 mutation carriers. Our results have important implications for the design of chemopreventive and therapeutic interventions in this high-risk patient population.
Bio: Dr. Subhajyoti De is an Assistant Professor of Pathology and Laboratory Medicine at Rutgers, the State University of New Jersey and a member of Rutgers Cancer Institute. Previously, he completed his PhD at the University of Cambridge, UK and postdoctoral work at Harvard University. His research group develops and applies genomics, systems biology, and computational modeling approaches to cancer.
Title: Image Analysis Methods for Measuring the Micro-Environment of Single Cells Within Three-Dimensional Multicellular Spheroids: Optimization for Breakthroughs in Drug Discovery.
Start Time: 14:00 PM
Speaker: Dr. Ty Voss, Voss Imaging Informatics (LLC)
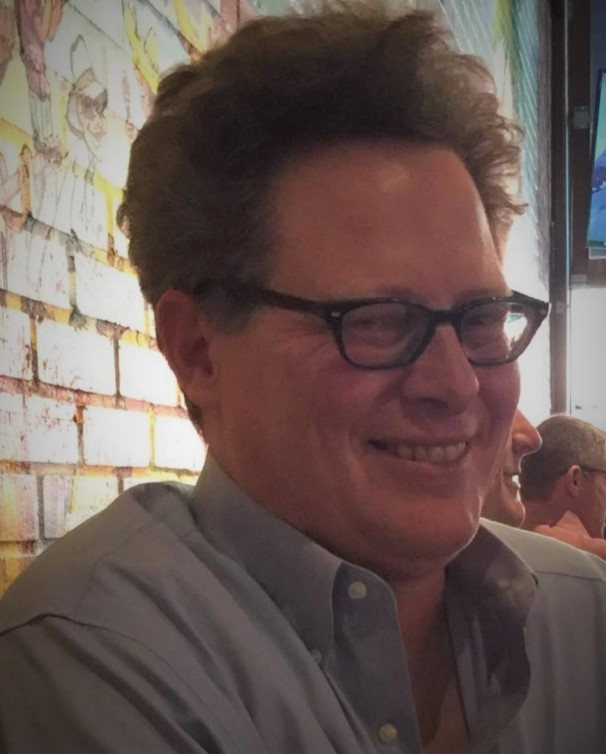
Abstract: The natural in vivo three-dimensional (3D) organization of complex tissues allows individual cells and collections of localized cell-types to signal and behave in ways that may not be possible when those cells are grown in mono-layer tissue culture. These 3D micro-environments are important for understanding diverse disease states including cancer, chronic wound healing, auto-immune diseases, and neuro-degenerative syndromes. Accordingly, the pharmaceutical research industry has recently committed significant resources towards measuring the behavior of multicellular spheroids/organoids in more high-throughput modalities for screening purposes. However, the majority of these published high-throughput imaging studies have only measured ‘whole spheroid-level responses’ and have lacked single-cell level information. In contrast, several academic studies have shown that single-cell level 3D imaging measurements can be obtained from organoid samples, but the image acquisition and analysis methods do not have sufficient throughput for screening purposes. In this presentation, we will describe our recent efforts to optimize sample preparation, high-throughput image acquisition, and parallelized automated 3D image analysis. This convergence of optimized methods provides single-cell level data from large numbers of spheroid samples, which will be essential for ongoing drug discovery screens.
Bio: Ty C. Voss received a BSc degree in biochemistry from Oklahoma State University in 1994 and then studied for his molecular biology doctoral degree at Tulane University. During this period, he began quantifying and computationally modeling the effects of hormonal regulation on transcription at the single cell level. He extended these studies and developed more advanced automated microscopy approaches during two fellowships, first at the University of Virginia and then at the NIH Campus in Bethesda MD. In 2009, He began collaborating with NCI Sr. Research Faculty to establish the NCI High-Throughput Imaging Facility (HiTIF). He served as the HiTIF head for four years, developing and executing automated microscopy assays, along with siRNA screening campaigns. In 2013, He founded a bio-informatics business, Voss Imaging Informatics (LLC), that provides consulting services and customized analytical software solutions for multiple government, academic, and commercial research clients. These contracted consulting projects are presently ongoing (2018). Dr. Voss also acted as Senior Applications Scientist for North America with the PerkinElmer Cellular Imaging and Analysis Team during 2014-2016.
Title: Life and death decisions- classification, characterization and predictions of death in neuronal models of neurodegenerative disease
Start Time: 16:30 PM
Speaker: Dr. Jeremy Linsley, Gladstone Institutes and the University of California, San Francisco
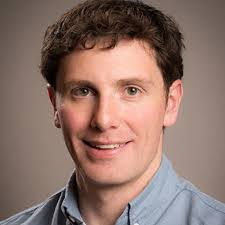
Abstract: Understanding the cellular changes that destine a neuron to live or to die is a fundamental challenge to understanding neurodegenerative disease. We use robotic microscopy, an imaging platform that uses automated identification and tracking of millions of individual neurons in culture over time, to quantitatively relate intermediate changes within a neuron to its fate. Interpreting changes within degenerating neurons is complicated both by the task of analyzing dense and complex microscopy images, as well as by the complication of analyzing progressive and often sporadic diseases. Using convolutional and recurrent neural networks, we quantify, interpret, and predict features in human patient derived neurons. These in silico methods reliably predict standard conventional cell-biological techniques, facilitate measurements that would be problematic or impossible to acquire using conventional methods, and outperform human manual curation. By surveying neurons derived from patients across neurodegenerative disease, we are developing algorithms for classifying unhealthy and diseased neurons across a variety of neurodegenerative diseases. Additionally, we are able to reliably predict the life or death of a neuron prior to death, focusing our window for understanding the mechanisms for these progressive diseases. We believe the use of these technologies will enhance our understanding of the pathology and physiology underlying neurodegenerative diseases with the goal of creating new therapies and cures for these devastating diseases.
Bio: Dr. Jeremy Linsley is a postdoctoral fellow in Steve Finkbeiner’s lab in the Center for Systems and Therapeutics at the Gladstone Institutes and the University of California, San Francisco. Jeremy studies neurodegenerative disease models using four-dimensional imaging, novel fluorescent biosensors, and deep learning analysis. He combines artificial intelligence approaches with a fully automated robotic microscope developed by the Finkbeiner laboratory that can track individual cells for hours, days, or even months. Using these tools, he is developing cutting-edge technologies to detect, predict, and analyze a range neurodegenerative diseases. By shedding light on underlying pathology and physiology, Linsley’s work aims to create new therapies and cures for these devastating diseases. Linsley earned a PhD in molecular and cellular biology from the University of Michigan, where he studied a family of adaptor-like proteins. He explained the mechanism of action for one of the proteins in skeletal muscle and discovered that a mutation in one of the proteins was the basis for the debilitating Native American myopathy disease.
Title: Efficient and Scalable Tools for Large Scale Microscopy Image Analysis
Start Time: 17:00 PM
Speaker: Dr. Erhan Bas, Janelia Research Campus of HHMI
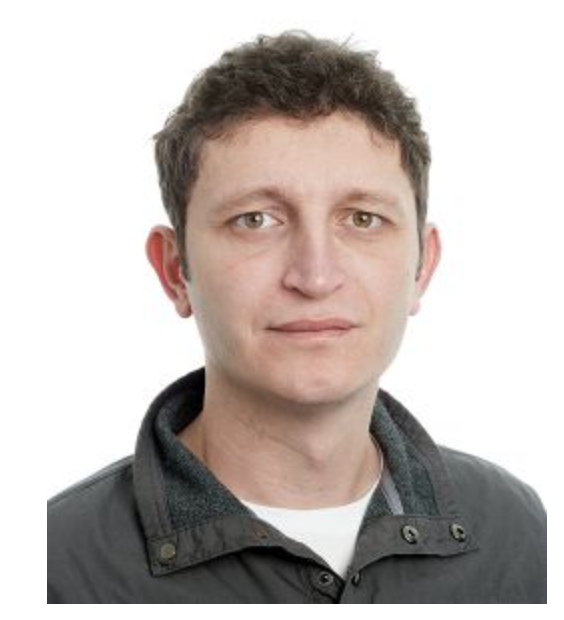
Abstract: With the advances in hardware and computational tools, large-scale high resolution volumetric imaging of whole systems is possible. Analysis of large volumes requires scalable segmentation algorithms and visualization platforms to efficiently stream through data and utilize user feedback. We developed an interactive segmentation and visualization framework for proofreading of large (tens of TBs) sparse volumetric datasets that aims to minimize user interaction with directed segmentation workflows. Developed tools enabled the analysis and proofread of more than 500TBs of volumetric data and resulted in the largest axonal reconstruction database available(https:http://ml-neuronbrowser.janelia.org/).
Bio: Erhan Bas received his PhD in Electrical and Computer Engineering from Northeastern University, Boston in 2011. He joined the Mouselight team at Janelia Research Campus (JRC) of HHMI as a computer scientist in 2015. At JRC, he is interested in large scale image analysis techniques for neuronal morphology analysis. Before joining to JRC, he was with the computer vision lab at GE Global Research in Niskayuna, NY, where he led a team of material and computer scientists working on industrial inspection technologies and he was an adjunct professor at Rensselaer Polytechnic University (RPI), Troy, NY where he taught Biological Image Analysis course. His general research interests include machine vision and statistical pattern recognition with various applications in biomedical and industrial image processing. He is one of the five Diadem challenge finalists and he serves as a member of IEEE Bio Imaging and Signal Processing Technical Committee, where he has been serving as an organizing committee member and area chair of ICIP 2015 and ISBI 2015&2018.